The "Business Intelligence vs. Data Science" debate is one of the hottest topics in analytics, and a common point of contention among data professionals.
A Data Scientist might argue that BI skills are obsolete, while an Analyst might claim that techniques like Deep Learning and AI will never become practical analytics tools.
So... who do you listen to?
If you want my advice, I say ignore both of them :)
The problem with these conversations is that they inevitably turn into unproductive debates, where people feel pressured to choose sides like it's some sort of zero-sum game.
Instead of arguing about the which path is "right" or "better", we should be talking about how BI and Data Science have many more similarities than differences, and how they can work together to accomplish incredible things.
Let's shift the conversation from BI vs. Data Science to BI + Data Science
Whether you're talking about data analysis, data science, machine learning, predictive modeling, business intelligence or any other "flavor" of analytics, it all boils down to the same ultimate objective: USING DATA TO MAKE SMART DECISIONS.
For those of you who appreciate analogies, consider the process of building a house. While everyone is aligned towards the same goal, there are individual stakeholders who each focus on specific tasks and deploy specialized skills to accomplish them.
For example, architects drive the vision and design, construction crews build the foundation and framing, plumbers and electricians make sure water and electricity flow to the right places, and designers and landscapers make the home user-friendly and visually appealing.
When you're building a house, it doesn't make sense to choose sides between the plumber and the electrician. Both play specific, equally important roles in the house-building process.
Similarly, it doesn't make sense to choose sides between Business Intelligence and Data Science, as both play equally important roles when it comes to extracting insight from data.
So if they're both important, when would you use one over the other?
The differences lie in the types of questions you're asking of the data and the types of tools you're using to answer them:

Let's break these comparisons down, in terms of objectives, tools, data and deliverables.
OBJECTIVES
Perhaps the biggest difference between BI and Data Science is when each approach should be used. This comes down to the objective at hand, and the specific question you seek to answer.
Business Intelligence is typically used for descriptive analytics, and understanding what happened, why it happened, and how we can learn from it.
BI often deals with specific, known questions, like:
Why are sales declining?
Which products drive the highest ROI?
Where are my most valuable customers coming from?
Generally speaking, the goal of Business Intelligence is to identify patterns and trends which can be used to derive clear, actionable insights and recommendations.
Data Science, on the other hand, is typically used for predictive and prescriptive analytics, and answering questions to help us understand what will happen in the future.
Data Science often deals with unknowns, like:
What's the likelihood of an employee quitting?
Which product will a customer purchase next?
How much will revenue increase next quarter?
The goal of Data Science is to test hypotheses through experimentation and iteration, and develop statistical models to help understand the world around us.
TOOLS
While there's certainly quite a bit of overlap, Data Scientists and Business Intelligence Analysts tend to lean on different types of tools to accomplish the objectives above.
BI specialists often use a "stack" of tools designed to capture, prep, transform, store, analyze, and visualize data. This typically includes web analytics tools like Google Analytics, database tools like SQL or Azure, ETL tools like Power Query or Alteryx, spreadsheet tools like Excel, and full-stack BI platforms like Power BI and Tableau.
Data Scientists tend to rely heavily on programming tools and languages like Python, R and JavaScript, which are designed for flexibility and open source collaboration. These tools excel at handling massive amounts of data, and identifying complex patterns and relationships which would be impossible to detect through visual analytics.
As the analytics landscape evolves, the lines between pure BI and Data Science tools are continuing to blur. Platforms designed for Business Intelligence now support AI/ML models, and languages like Python are playing a bigger role in the BI workflow as they become more approachable to non-coders.
DATA
We live in a world where data is EVERYWHERE.
While volume and velocity continues to grow exponentially, the sheer variety of data is changing as well. While the term "data" was once reserved for numerical values, now it can be applied to virtually anything that can be analyzed: text, audio, video, images, IoT signals, etc.
Business Intelligence tools and techniques are generally designed to deal with structured data sources, like tables of data or relational models containing intuitive dimensions and metrics (product info, sales records, customer databases, etc).
Data Science tools are typically better suited to handle high-velocity, unstructured data, as they rely on math and statistical modeling (vs. human intuition or visual analysis) to process large amounts of data, identify complex interactions, and convert abstract data formats into model-friendly features.
DELIVERABLES
Last but not least, let's compare the outputs or deliverables commonly associated with Business Intelligence and Data Science projects.
On the Business Intelligence side, deliverables tend to be visuals, reports, dashboards or tools, designed to create data-driven narratives, communicate key insights and business recommendations, or provide end users with interactive tools for data exploration or ad hoc analysis.
For Data Science projects, deliverables tend to be statistical models or predictions, which have been trained and optimized to answer specific questions. This might include a logistic regression model used to flag fraudelent transactions, a decision tree used to predict subscriber churn, or a set of customer segments derived from an unsupervised Machine Learning model.
BI + DATA SCIENCE: BETTER TOGETHER
If there are only two things you take away from this post, they should be:
BI & Data Science are two players on the same team, and serve equally important roles in the broader analytics world
While there are differences in objectives, tools, data and deliverables, the lines between BI and Data Science are blurring every day
If you are an aspiring analytics professional trying to find your path, remember that there are no strict "rules" here.
A Business Intelligence Analyst might build predictive models to forecast profit margins, or write Python code to scrape data from the web. Similarly, a Data Scientist might design a Power BI dashboard to track business KPIs, or use Excel for ad hoc analysis.
My advice? Ignore the labels and create your own path. If you love working with data and seek out projects that challenge and inspire you, you can't go wrong.
Happy analyzing 🤟
Up to 50% Off Maven Pro Plans
Spring Savings Sale
Take advantage of this limited-time offer and save up to 50% off unlimited Maven access!
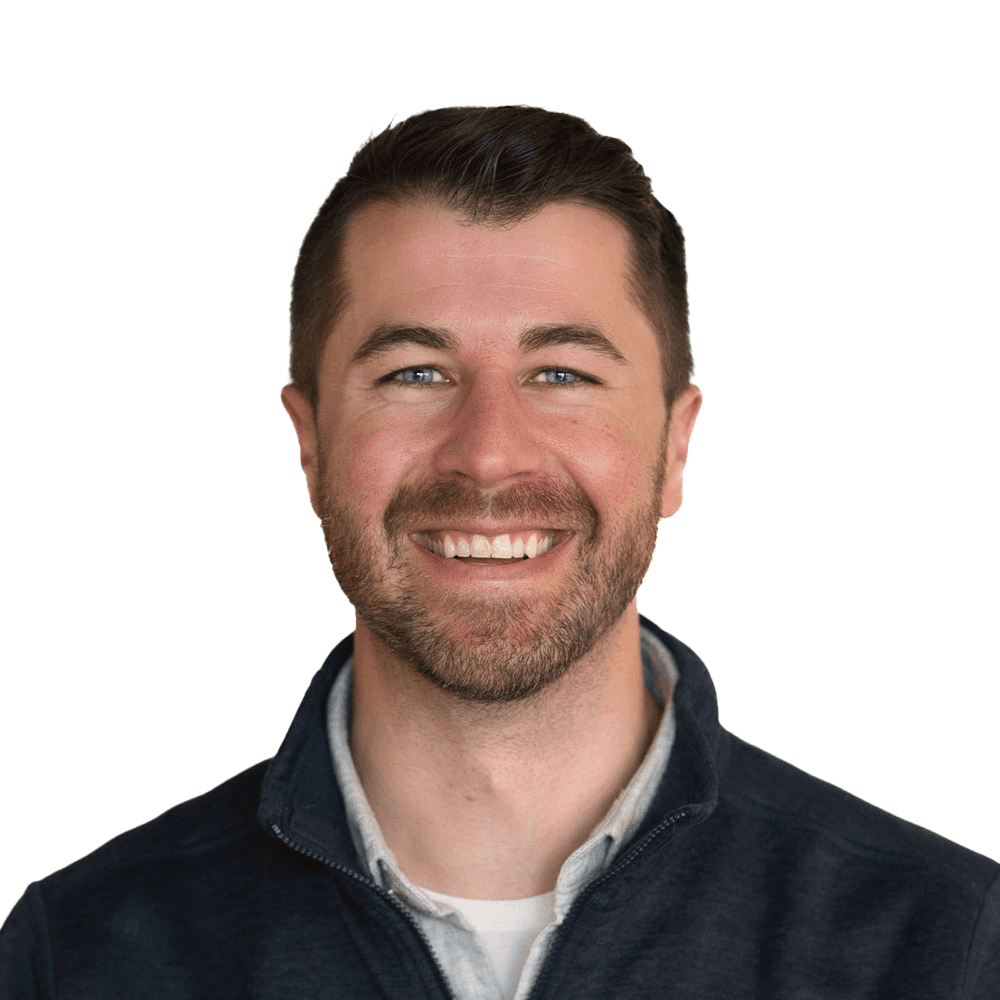
Chris Dutton
Founder & CPO
Chris is an EdTech entrepreneur and best-selling Data Analytics instructor. As Founder and Chief Product Officer at Maven Analytics, his work has been featured by USA Today, Business Insider, Entrepreneur and the New York Times, reaching more than 1,000,000 students around the world.