If you’re trying to launch a data career or are just starting out, you might have some questions about the different things analytics professionals actually do.
Well, that’s exactly what we’re talking about today.
Here are 10 examples of ways analystics pros can add value to a business...
1. Paid marketing channel optimization
We are starting off here because this is one of my all-time favorites. Any company that is doing paid marketing needs strong data people in order to do it well. If you’re strong here, you can make a huge impact, and that impact can be extremely visible. Remember, when you can produce revenue, even the CEO takes note of your work.
The first task channel analysts will focus on is just making sure the data infrastructure is set up so you can see what’s going on. You’ll ask questions like…
How much money are we spending?
How much revenue is that spend driving?
That’s the basic idea. Pretty simple, right?
Note that in some cases you’ll be looking at something other than revenue as a success metric. That could be new signups, B2B leads, white paper downloads, etc. But the idea is the same... What is the cost of this marketing? And how much of our positive outcomes are we driving with that cost?
Next, you will apply this same logic to all sorts of segments within your marketing to optimize it. You'll be trying to figure out which pockets of spend are more or less efficient than the others, so that you can lean into the good ones and then either fix or discontinue the bad ones.
Is Google more efficient than Instagram? Maybe we should shift spend to Google.
Is the new video ad driving more sales per dollar spent than the old image ads?
Which keywords have the best return on marketing spend?
Which Facebook targeting method is driving the most spend? Is that spend driving signups efficiently?
How are the new TV ads performing?
None of this stuff is overly complicated. You just need to know some basic math (fractions), understand the marketing channels being discussed (so you can speak the channel manager’s language and understand the levers they can pull), and know how to get the data and slice and dice it.
Personally I find this type of work to be one of the most fun things an Analyst can work on because when you are good at it, you can really juice a company’s revenue performance, and that’s a very visible thing. It also feels really good to be contributing in this way. When you drive more revenue, it helps the company pay all of your coworkers, attract new talent, etc. Being a rainmaker is really fun.
Because this work involves handling a number of different data sources (for example: Google ad spend, Facebook ad spend, TV ad spend, and signups or revenue driven by ads), you’ll need to aggregate all of it for analysis. Excel is one of the most common ways to tackle the aggregation exercise, especially in the early days. Then as you get more mature and are sure you’re going to be investing in the channels long term, you may look to automate some of the data aggregation work using tools like Python, SQL, Power BI and Tableau.
Let’s discuss a specific example to make this feel more concrete…
I was running Marketing for a startup that had about six different paid advertising channels. I was a one person marketing team, initially doing all the data aggregation and analysis in Excel. As our campaigns got more mature and more complex, I decided I wanted to automate as much as I could. With the help of one of our Engineers, we automated the data aggregation work. And then I wrote some automated bidding algorithms for the repetitive daily/weekly optimizations. Here’s what we did to pull that off…
Set up automated CSV reports from the various ad sources (Google, Bing, Facebook, etc) using their reporting tools, which were emailed to reporting@company.com (not the real domain) every morning with the data from the previous day.
Used Python to run a daily job that grabbed those new CSV files from the email, parsed the data, and inserted it into a central SQL database (which already contained our revenue data).
Wrote some bidding algorithms to analyze the various spend data to find pockets that were efficient or inefficient, and spit out marching orders like “bid up this keyword by X%” or “remove this ad unit”, etc. Once these were written, they were put into a SQL stored procedure so it was super easy to run them regularly. From time to time we would tweak the bidding algorithm as we learned more about our campaigns, but mostly this was on auto-pilot so we could focus on other areas.
Hopefully this example helps. It was a really fun project and it also let us manage a large amount of marketing spend effectively with an extremely small team, which was great for our business.
2. Website analysis and optimization
Another really fun area that data people can contribute quickly in almost any business is by using their data skills to analyze and optimize website performance.
First, the task is just to understand what’s happening on your website. You’ll ask…
How much traffic is the website getting? Is traffic volume growing?
Where are visitors coming from? Which sources are driving them?
What pages are our visitors landing on? Homepage? Blog? Something else?
What does typical behavior of a visitor look like? Do most people bounce off? Or does the website do a great job converting traffic to purchase?
Are there particular places where customers drop off in the purchase flow?
If you can get good at answering questions like these, then the next step is pretty obvious… use your insights to improve the business!
Here are some examples of things that you could look for to add value…
Is there a blog post that’s doing an amazing job of getting SEO traffic and driving revenue? Point it out and suggest similar topics to copy its success.
Are there particular spots where people abandon your site during the checkout process? Make sure the website manager knows about it, and offer thoughts as to what might be causing dropoff and proposing some potential solutions to improve things.
Are there any parts of your website that just aren’t getting much traffic at all? Maybe they are hard to get to? If not, maybe they just aren’t interesting to customers and you could use their real estate for something else? Again, let the website manager or eCommerce owner know what you’ve found and come with suggestions for improvement.
If you can provide insights like this, you’re sure to help your business improve continuously.
To pull out these insights, you’ll need to get good at using web analytics tools like Google Analytics, Adobe Analytics, Mixpanel, etc. If you want to learn, check out Google’s free training for Google Analytics. Their video series is great, free, and their platform is the most widely used, so your skills will be very marketable.
Again, web analytics is an area I have personally found to be a ton of fun, and one where you can produce high visibility results for your business.
3. Customer analytics
Customer analytics can be another really fun area where analysts can help a business.
You might be looking for specific customers who are particularly high value clients, who may warrant special treatment or offers to retain them.
You could be doing segmentation analysis to group customers into different buckets based on their attitudes or purchasing criteria. For instance, I used to do some work for a major cruise line, where we created different customer segments in order to understand the types of offers they might be most interested in. Some customers were very value oriented, and we would offer them really affordable discount rates on the least expensive rooms. Others were extremely affluent and responded well to deals on the fanciest rooms we had to book, or adding free shore excursions.
Another type of customer analysis is looking at customer behavior for brand new customers and trying to understand how valuable we think they will be based on their early actions. This may get into a gray area that gets closer to financial analysis or predictive modelling (welcome to data careers. The roles can be murky!).
You may find yourself analyzing different cohorts of customers over time, to understand whether you’re acquiring more customers as your business matures, and how the new cohorts are performing vs prior cohorts of customers.
If you’re doing this type of analysis, you’re likely using a lot of internal tools to get your customer data. The data tools you could be leveraging may run the full spectrum of Excel, SQL, Power BI, Tableau, Python, R, etc.
4. Financial analysis
No surprise almost any serious business is investing some sort of resources into financial analysis. You need solid data people to work on this stuff.
Here are some of the things those data people could be working on…
Looking at cost drivers. Are there places the business could be more efficient?
Looking at revenue drivers. Where is the revenue growing? How can we do more of that? (some overlap with marketing analytics for sure)
Building forecasting models to understand what the business will look like in the near-term or mid-term future.
Looking at the financials of similar companies as acquisition targets (larger companies will do this. Not applicable at small shops)
Working with company financial statements (balance sheets, cash flow statements, income statements)
The types of tools you’ll be using for this type of work can vary depending on exactly what you’re doing. One thing is universal though, business acumen is absolutely crucial in this area (it is for most other data roles too).
5. Product analytics
Software as a service companies always need data people who can dive in and figure out how their customers are using the product.
They’ll ask you to understand behaviors, and mine for insights that will help them keep customers longer and find and attract new customers.
You could be looking at any of these areas below, or more likely all of them!
For B2B software, can you identify certain power users within each of your customers’ teams? What are they using the service for? This can help you try to convert more current users to power user status, and can also inform marketing and sales about the most valuable features.
For either B2B or B2C products, what does behavior look like during the onboarding process? Are customers interacting with your most valuable features? If not, why not? If you can paint this picture for Product Managers, it can help them think about activation and onboarding and getting the most useful features prioritized so that users are more likely to stick around.
What does the usage of your product look like over time? Is there a “honeymoon phase” where users are extremely engaged, and then drop off after? Does usage of your product build over time? Is it more steady?
There are lots of different tools you might use for this, which will depend on the company. My personal favorite has always been re-purposing the production database as an analytics tool by creating a read only replica for analysis. The idea here is that you’re already using a SQL database to produce your software application (most back-end systems use a relational database to house the data used to provide the experience). Since that’s already there, if you know how to use SQL for analysis, you can answer almost any question you can come up with, without having to try to implement additional analytics tools. Not only is this easier because it doesn’t require implementation, I also find the range of questions you can answer to be superior when you are using a SQL database compared to an off the shelf drag and drop solution. The downside is, you need to know SQL for this. Personally, I would say if you want to be a product Analyst, you should learn SQL. Without it, this particular flavor of analytics is very difficult to be great at, while others you could do fine with just Excel or Excel and Power BI or Tableau.
One of the most fun roles I ever had was doing a lot of product analytics. It was at a startup that was growing really fast, had tons of data, but didn’t really know how to use it until I got there. So there was SO MUCH work to do. I never had the chance to be bored, and got to prioritize the most impactful projects. That was really fun.
6. Leading the experimentation function
This one made the list because again, it’s one of my all-time favorites.
When I say experimentation, I’m talking about running simple A vs B split tests on your website or product application, to try and drive more of some desired outcome to make your business better. That outcome could be using the product more (engagement) or you could be trying to test website pages that will directly drive more revenue for your business. In either case, you’re typically coming up with new versions for a given page, screen, or email campaign, which will perform better than what you’ve got there currently. Instead of just relying on your opinion or that of the Designer, you set up a split test and let real performance do the talking. Send 50% of your users to the current one, and 50% to the new one. You’ll be looking at conversion rates to see which version performs better.
The concept is fairly simple, and the conversion rate calculation is fairly trivial too… looking at how many people convert to your happy outcome out of how many are exposed.
Here is an example…
1,000 people saw page A and 10 of them converted to purchase (1%)
1,000 people saw page B and 50 of them converted to purchase (5%)
In this case, we would say page B does a better job at converting our users to the happy outcome, so we would end the experiment and roll this out to all of our users so that we can make more money,
While this may seem pretty simple, a lot of non data people get intimidated by some of the nitty gritty details…
proper experimental test design (random assignment, concurrent flighting, etc)
pulling the data (where to get it? Is it correct?)
understanding statistical significance (when to saw the differences are big enough that we can say they are “real” vs “normal random chance variation”)
Even though each of these areas may seem fairly basic, and certainly something non data people could learn in short order, we typically find that they don’t, and instead most companies handle this by putting a data person in charge of experimentation.
Again, this is a great example of somewhere that a young analyst can make a major impact, especially if you get the chance to run experiments on mission critical areas like your checkout flow or high traffic landing pages.
The tools you’ll use for this one are going to be things like Optimizely, Google Optimizer, LaunchDarkly, SiteSpect, or maybe a home-grown experiment platform. You’ll often be looking at data in Excel, or maybe pulling some of it from your SQL database. And for statistical analysis there are some great free calculators on the internet, or you can also build your own with formulas in Excel.
7. Predictive modeling
This is getting more into the Data Scientist realm. We had to include something here, because this work is really fun!
Let’s jump into some concrete examples to start talking about this type of work…
You could create a model to understand how valuable new customers are expected to be based on their initial behavior.
Another valuable modeling exercise might be to understand different features of your product where interaction predicts longer customer retention and higher lifetime value.
Companies like Amazon and Netflix analyze past purchase or viewing data to make predictive recommendations for which products or shows you may want to purchase or watch next. If this is done well it leads to a better experience and more money for the companies.
These are just a few examples of where Data Scientists can add value to a business with predictive modelling work.
If this sounds fun to you, you’re likely going to want to learn Python or R (my choice is Python), and you’ll want to study statistics, and start to develop some solid business acumen (which is true for any data role).
8. Subscription analytics
Subscription analysis applies to companies where customers sign up and stay signed up month to month or year to year with an ongoing subscription model.
This can be great for businesses, who create streams of revenue that grow and grow, especially if they have smart data people who can help them do that.
Here are some of the things you might be digging into as an Analyst in a subscription-based business…
How many new subscribers have we gotten in the last month?
How many previous subscribers did we lose during that period?
How much monthly revenue are we making from subscriptions?
What is the typical lifetime value from a subscriber?
Is lifetime value going up or down with new cohorts of subscribers?
What percentage of our subscribers take a monthly plan vs a discounted annual plan?
When subscribers leave our business, what are the most common reasons? Is there anything we can do about that?
Are there certain actions that customers take which make them “power users” or predict that they will stay around longer?
If this type of analysis sounds fun, you’re in luck, as you can slice and dice data with any of the usual suspect tools… Excel, Power BI, Tableau, SQL, Python, R, etc. Personally, I have found SQL skills to be the most useful here, because of the flexibility and your ability to ask extremely granular questions about customer behavior and then roll them up to whatever higher level you are interested in.
9. Manufacturing analysis
This is an area I have never worked on personally, but I used to work alongside a group of analysts who did this type of work for a large international manufacturer.
Here are some of the things they were working on analyzing…
Which products have the best margins?
Which components of our goods generate the most cost? Are there ways we could reduce cost?
Are we staffed appropriately at the plant? Are we able to keep up with demand surges? Do we have any periods of very low employee utilization?
Which products tend to have high refund or defect rates?
For products with high refund or defect rates, what types of complaints are customers filing? What can we do to fix this going forward?
How well are our suppliers delivering for us? Are their shipments typically on-time? Are the orders delivered correct? Are certain suppliers cheaper? Do certain suppliers seem to have lower refund rates?
These are just a few examples. Again, I never did this work myself directly. But I did get to see folks who were doing it regularly. They seemed pretty happy.
10. Building data tools to enable others
This last one is a bit different from the rest. With all of the other areas we talked about, the Analyst was diving into the data and searching for actionable insights themselves. However, sometimes instead of doing the analysis yourself, you’ll use your data skills to create tools or dashboards that will enable others to find their own actionable insights or do their jobs better.
That might be using Power BI to create an automated dashboard that will help someone track their website performance.
Or you might create an Excel-based tool that will allow someone less data savvy than yourself to do some simple analysis using drop-down filters or pivot tables.
You might use a tool like Metabase or Tableau to create dynamic reports on top of underlying SQL code so that folks who don’t know how to use the SQL database can still get access.
Whatever you end up doing here, the common theme is that you’re using your data skills to get data into the hands of others. This is pretty cool, because you’re leveraging yourself. You’re letting your data brain spread around the organization by creating these tools and making others better with data.
Wrapping up
Hopefully seeing all of these examples made this more concrete and helped you understand some of the day to day projects real analysts might work on in different types of roles.
It's important to note these are just examples. There are LOTS of other ways us data people can help a business that didn't make this list.
Sometimes you might find yourself with your work fitting neatly into one of these categories, or a different category not listed here. Other times, you might find that your role spans lots of different types of work. These types of roles have been my favorite, because the work never seems to get boring. There is always something new to learn. If you made it this far, thanks for reading. Hit me up on LinkedIn and tell me what your ideal role would look like.
Happy learning! -John
Ready to get started
Sign up for FREE today and level up your data skills
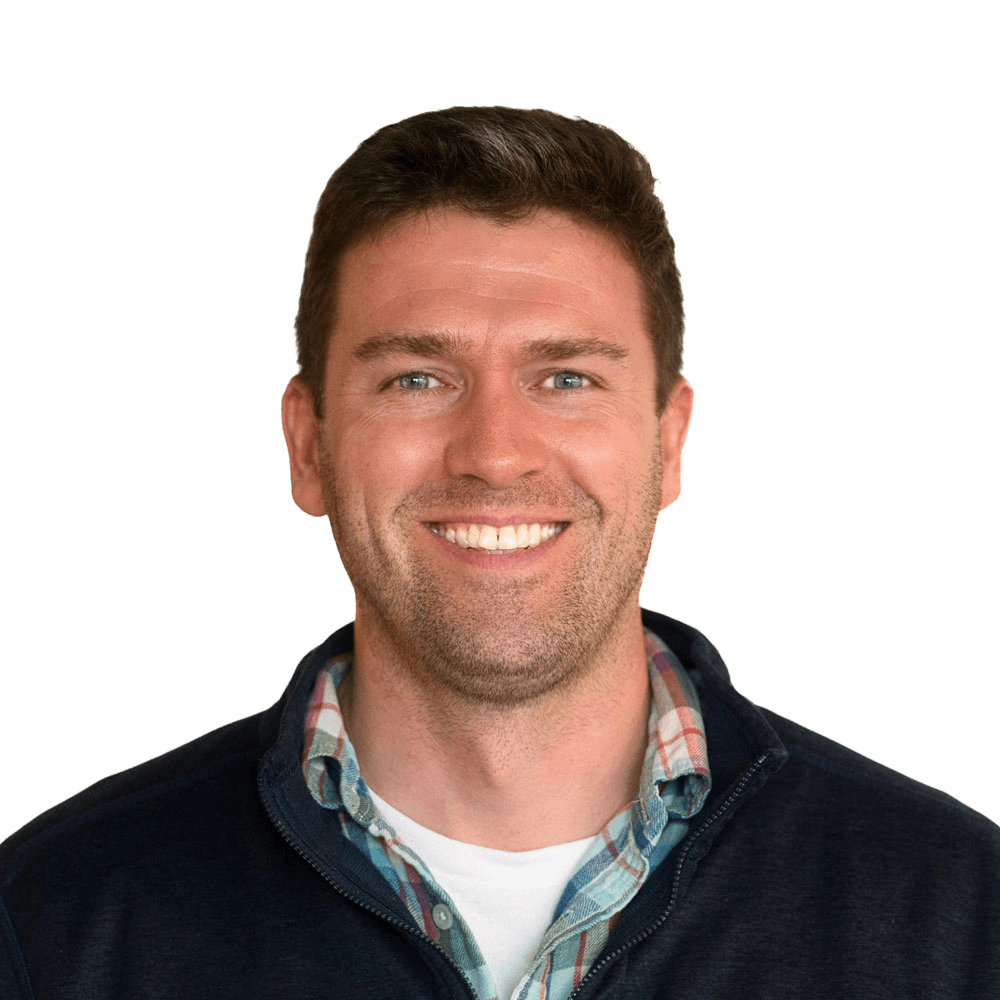
John Pauler
Partner & CRO
John brings over 15 years of business intelligence experience to the Maven team, having worked with companies ranging from Fortune 500 to early-stage startups. As a MySQL expert, he has played leadership roles across analytics, marketing, SaaS and product teams.