Are you trying to launch a data career in 2022?
If you are, this one is for you.
We'll cover a simple roadmap that will how to get a data analyst job in 2022.
Here's your framework for landing your dream role this year...
Make a plan
Explore potential paths and pick one
Practice using the tools
Learn how to think like a data person
Build a project portfolio
Get good at marketing your skills
Master the data analyst interview
Sound like a reasonable course of action?
Great :)
Like I said before, this roadmap is fairly simple, but that doesn't mean that it's going to be easy or that you'll get where you want to go by the end of this week. Pulling this off takes dedication to your plan, and consistent effort.
If you commit to doing this, you WILL succeed.
Now let's get into the details of each of the steps you'l be taking...
1. Make a plan
This part is important, but it's also dangerous. We're naturally analytical people. There's both good and bad that come along with that.
The good? We'll take in all the data available to us, hopefully use it well, and make smart, fact-based decisions.
The bad? We tend to overthink, and this can lead to analysis paralysis. We keep mulling things over, and searching for new information, and this eats up a ton of time with no action being taken.
Make a plan that gets you through these 7 steps, and don't spend more than 1 hour.
If you're spending more time than that, you're doing it wrong.
The goal here is to have a rough plan of attack, ideally written down (this will help you stick to it) and then to get moving ASAP.
It doesn't have to perfect, or even close. It just has to be decent, and something you can get started on. You can, and likely will, change some of the details as you work through it.
If you like the plan I'm laying out for you here, you can use this. If you want to make your own, that's great too.
Just make sure that you have a plan, it covers theese 7 steps, and don't spend more than an hour. Don't overthink. You've got work to do!
When you get to the end of this article, I'm going to ask you to write down your specific plan. Writing it down really is critical. When we put pen to paper, it makes us more likely to follow through.
2. Explore potential paths and pick one
This is going to be a major step toward orienting yourself on your path.
Your goal is to just generally understand the different types of career paths that are out there in data, so that you can start making moves towards the one or two that sound most exciting to you.
It's extremely important to stress again, that you should not overthink this. Your goal should be to get a quick understanding of the difference between Data Analyst, Data Scientist, Data Engineer, and Database Administrator.
Aim to spend something like 2 hours tops, getting your head around these roles, and thinking about about the one or two you want to go after. Again, as you progress throuh your plan, you may decide to pivot. That's okay. You just need to plot a course and start taking action today.
I'm not going to spend time here explaining the differences between the roles and going into depth about how you should decide on a path.
Chris already put together an awesome guide on this topic:
Finding Your Analytics Career Path
You can also Google these roles. There are lots of great resources out there.
When you have a decent understanding of the various paths, pick your top choice, and a second choice. Once you've done this, you're ready to move on to step 3, where you'll start learning the tools of the trade.
3. Practice using the tools
In 2022, having some exposure to the tools you'll use in your chosen data career is table stakes if you want to get hired.
Your competition is already using the tools, and working on relevant projects they can discuss with employers. So you need to be doing that too.
Quick note here, as you start tackling step 3 of your plan, you'll should keep steps 4 and 5 in mind at the same time, as you can and should develop these in parallel...
Practice using the tools
Learn how to think like a data person
Build a project portfolio
As you're working on learning the tools, aim for hands-on learning, with projects that are relevant to work you're hoping to do professionally.
If you pick the right types of projects, as you start working with the tools and relevant data sets, you'll be practicing answering real business questions and learning how to think like a professional analyst. You will also be able to package up these projects into an Analytics portfolio which you can use to attract job opportunities and discuss your experience with potential employers.
We'll cover steps 4 and 5 more below, but for now, just remember we want you tackling them in parallel as you make progress on step 3.
Here is how I would tackle learning the tools (we'll explain this in more detail too)...
Everyone
Excel
SQL
Data Analysts
Power BI (or substitute Tableau)
Python
Data Scientists
Python
R
Power BI (or substitute Tableau)
Data Engineers
Python
Hadoop/Apache Spark
Cloud tools (AWS, Azure, etc)
Alright, let's discuss the details here...
I say everyone should start with Excel, and I mean it.
First, Excel is EVERYWHERE. Basically every company you will ever work for will use Excel or Google Sheets (often both) and you will encounter data that is stored in Excel files, or handed to you in Excel files after being output from some other system. When you're handed an Excel file and just need some quick information, loading the data into a SQL database or opening up Power BI is a little overkill. Excel remains powerful, and for many tasks, it is the fastest and easiest tool.
Next, Excel is the easiest of these tools to learn, and a great "gateway" which will help you pick up the other tools later. With Excel, you get great exposure to row and column thinking. You can easily work with your data, manually editing values and applying filtering criteria. It's also a great exposure to logical operations, which you can practice by creating formulas to calculate new values based on your existing data.
Next, I say everyone should learn SQL.
Yes, I'm biased because this is the tool I'm personally most passionate about. But there is good reason for my bias :)
Check out some job descriptions for data roles. You'll see a pattern... most of them list SQL skills as a core requirement.
Why is that? Why does it seem like almost every data job these days needs SQL skills? Well, it's just the way companies store data. Systems are built to use relational databases to store tons of information on everything from financials, to customers, to manufacturing, to operations, and everything in between. And SQL is how we access that data.
Want to be a Data Analyst? You should learn SQL.
Data Scientists... you need to learn SQL too.
Data Engineers? Yes, you definitely have to know SQL.
If you want to work with data, and you want to unlock more opportunities for yourself, SQL is one of the best things you can do. The demand for serious talent is still much greater than the supply, so if you become strong here, employment tends to be very easy to come by.
It's also not that painful. I'm a non-technical person myself. I taught myself SQL over ten years ago, and now I use it on the job every day.
It's a fairly intuitive language, which becomes pretty fun once you get the basics.
Alright, so everyone needs to know how to use Excel and SQL. What's next?
For aspiring Analysts, I recommend in most cases you go after Power BI next. Pairing Power BI data viz chops with your SQL skills is a serious 1-2 punch that from my perspective is one of the best ways to become valuable to an employer. The tools play well together, and when you can use SQL and Power BI together effectively, putting together an extremely strong portfolio project becomes much easier.
Why Power BI and not Tableau?
Great question. Tableau is awesome too. It also plays well with SQL. Historically, Tableau's data visualization tools are even a little better than Power BI's.
Wait... is this guy selling Tableau now?
Nope, stick with Power BI in 2022. Here's why...
Power BI's tools have come a long way, rapidly closing the Tableau gap and bringing most features up to parity
Power BI is cheap. The barrier to usage is low (for companies too! This is the part that really matters to you)
Power BI has taken market share recently, fast
Because Power BI has penetrated the market so quickly, and because it's still the relatively newer tool, there aren't enough true experts yet
All of this leads us to the key point... there are more opportunities for Power BI roles than there are talented people right now. So if you develop great skills here, you'll put yourself on the right side of supply and demand, and boost your chances of becoming employable quickly. At Maven, we are seeing lots of interest in Power BI talent at the moment. Become that talent the market is looking for!
When would I recommend you learn Tableau (or Looker, etc) instead?
If you already work somewhere that uses Tableau, and learning the tool would get you access to their data, that's what you should focus on. Always go with the tool that gets you immediate access to the data. This will lead to hands-on practice while you're on the clock. A lot of the skills are transferrable between different data viz tools too.
Before we get off Power BI, you may have noticed I added this to the bottom of the Data Scientist list too.
Yes, I do think Data Scientists should know the basics of Power BI. There are a number of reasons for this...
sometimes it's the easiest way to access a company's data, and you just need to do some quick and dirty visualization work
maybe you want to automate the reporting from a project you've put together. If your company uses Power BI, this is a really great tool for spinning up reporting and publishing it for others to use.
adding Power BI to your stack can turn from from 'just a Data Scientist' to more of a 'Data Scientist / Data Analyst' hybrid... talk about value and flexibility!
Next, let's talk about Python.
Python is critical for those aspiring to Data Science roles, and a real nice to have for Analysts.
If you want to be an Analyst, I would prioritize Python AFTER Power BI.
If you want to be a Data Scientist, it comes right after SQL, BEFORE Power BI.
So why Python? Why not prioritize R first? What's the big deal?
It's the same answer as the Power BI vs Tableau question... both tools are awesome, powerful, and extremely important if you want to market yourself as a Data Scientist. Python is just more in demand right now on the job market, so that's where you want to focus first.
For those who want to be Data Scientists, you should learn BOTH Python and R. The tools can work together, and knowing them both really paints you as a Data Scientist, and will maximize the breadth of your marketability to various organizations.
For Data Scientists, learn Python and R before Power BI (which is more of a nice to have).
For Data Analysts, learn Power BI first, then Python.
For those thinking about some sort of Data Analyst - Data Scientist hybrid skillset, learn them all :)
Just know this is a lot of material to cover, so you'll need to be smart about how you prioritize things. Use the lists above to build your plan.
For an even more in-depth look at the analytics tools landscape, and why Maven settled on the tools we've decided to teach, check out Chris' article:
Alright, before we move on, how should you go about learning all of these skills?
First, you don't have to pay to learn any of this stuff.
Wait what... he's not saying buy Maven courses?
That's right. There are tons of ways to learn these tools, for every budget and every learning style.
Some folks do really well with fully self-directed study. If you're someone who does a great job researching the topics, would feel comfortable building a full curriculum for yourself, would hold yourself accountable on your own, and want to keep your learning budget on the floor, then you could learn all of this stuff with YouTube, and never spend a single dime.
Just make sure you read Chris' article talking about self-directed learning before you fully commit to going off on your own:
Four Flaws of Self-Guided Learning
For those who want a little more structure about what you should learn within each tool set, there are some extremely affordable courses that give you a high quality education at a great price. Sometimes just having a defined curriculum to guide you through your journey can make these worth it over the fully self-directed option.
Yes, Maven offers a lot of these courses (I wish we taught Python, maybe we will soon), but you don't have to go with Maven. There are a number of great providers in the low cost self-paced training market. As long as you go with a training provider with a great reputation, you'll probably be in good shape.
Ideally look for these attributes in a self-paced course provider...
Lots of happy students
Ability to get help when you're stuck
A focus on project-based learning that is relevant to job roles
If you're someone who can commit to holding yourself accountable, following a plan, and you just want a nice structured curriculum laid out for you, then self-paced learning can be a great option. And it can be pretty inexpensive.
One more option is joining a bootcamp... this is going to be the most comprehensive learning experience, with instructors guiding you through everything, holding you accountable, helping you with your resume, your online profile, job applications, and helping you prepare for interviews to launch your data career. The downside? This is the most expensive option. Even the most affordable options start in the multiple thousands of dollars range, which is definitely not the right fit for everyone.
If you're considering investing in a bootcamp, check out this guide to make sure you're asking the right questions before you commit:
How to Choose the Best Data Analyst Bootcamp
Which type of learning is right for you?
It all depends on your own personal needs and learning style. Just be realistic about what will work best for you, commit to learning, and take action. That's the most important thing.
How long will this take?
Months to learn the basics, and a lifetime to master.
Remember, I said this was simple, but not easy. This is the part that's a lot of work. It's also where most people fail to put in the effort. So if you can commit and start learning, you'll be ahead of the pack.
If you focus on the right things, learn the tools effectively and practice with hands-on projects that you can use as a portfolio to market yourself, then you'll be able to start applying for jobs within a few months.
Then, commit to lifelong learning.
I've been doing this stuff every day since 2007, and still have tons to learn :)
4. Learn how to think like a data person
This is one that generally comes with experience, and develops over time with more and more practice.
As you expose yourself to more and more relevant projects, you'll learn how to ask the right questions, what to look for in the data, and you'll develop your intuition and business acumen.
There are no real silver bullet shortcuts here, but I would recommend some reading material that should help you develop a mental framework that will accelerate your learning as you work through real-world projects:
How to Build a Measurement Plan
10 Areas Young Analysts Can Add Value
Give these a read, and think about how you can apply what you've learned as you are working through your data projects.
5. Build a project portfolio
I cannot over-emphasize how important putting together a project portfolio is if you want to get hired for a data role in 2022.
Yes, you're going to be learning the tools of the trade. But so will your competition. So it isn't enough to just list your skills on a resume or on your LinkedIn profile.
If you want the job, you need to SHOW employers what you can do.
When you build a strong portfolio, you are creating an opportunity for employers to see your work in action. This is something you can discuss with them during the interview, and even walk them through your projects.
If you market your portfolio correctly, make it publicly accessible, and promote your work well, this can even lead to some passive discovery, where employers will start reaching out to you about potential opportunities.
Clay talks about how important putting his work out there was for his career pivot, and you can see his his portfolio site here.
Don't worry, you don't have to build your own website to do this effectively. Consider that a nice to have.
Ahmad talks about getting active on LinkedIn and how it completely changed his career trajectory.
What do these two have in common? They worked on relevant, real-world data projects, and they put them out there for the world to see. And this is the reason both of them hit an inflection point in their journey, and are thriving today.
Here's how you can take action to build a solid project portfolio to market your data skills as you build them...
1. Work on real world projects
Hopefully, the courses you are working on to learn data skills will be project-based. Start with these projects as you're building your portfolio.
Additionally, look for other data sets that you're interested in, and start diving into them to learn.
Any of these will be great options, and I recommend you exploring them all when you want new data sets.
The benefit of Kaggle and Data.World is they are massive, with more data than you could imagine or ever need. The downside... it can be overwhelming.
The Data Playground is a bit more niche, with data sets geared towards beginners and intermediate Analytics pros, and including analysis prompts to get you started.
Again, don't overthink... just find some data that's interesting, and start working with it. Progress is king!
After you've finished working with a data set...
2. Package your findingsHere's what you should focus on...
Summarize the business problem you tackled (1-2 sentences)
Summarize the key insights you found (1 sentence each, aim for the 3-5 most interesting things you found)
Include a strong data visualization. This makes your project eye catching to your audience, and shows how you tell a story visually
Include a technical flex if applicable. This is where you'll include your SQL or Python code, complex Excel or Power BI Workbooks, etc.
As you're packaging up these projects, I recommend you follow the order bulleted above.
If you go out of order, here's the problem...
Starting with a technical flex like SQL or Python code too early immediately narrows your audience and people lose interest before they get sucked in. Remember, you need to appeal to the data people, but also to recruiters, and functional leaders who aren't as technical. Don't get too in the weeds, or you'll lose them.
The first step is to hook them with a clear and concise description of the business problem you solved. For example...
"I used 1,000,000 rows of keyword data from Google Ads to optimize company XYZ's marketing budget and increase sales by 30%"
When you're writing this takeaway, talk about it like it's a real company. You want this to feel as concrete as possible, so the reader can understand the value you bring to the table with your skills, and how it will apply to their business.
After you've described the key business problem, go one layer deeper and summarize the key insights you found in the data. Again, be clear, concise, and try to make this feel as real and concrete to the reader as possible. You want them picturing getting insights like this delivered to them on the job, and thinking "wow, this person could really help my business".
Next, include the data visualization. This is both for the real value that great data storytellers can bring with strong visualizations, and to give your project that "eye candy" factor that will help it cut through the noise and draw your reader in.
After you've got your business problem, your insights, and your data viz, now and only now should you think about including your technical flex. This can be in the form SQL code, Python code, Power BI DAX code, impressive workbooks, etc.
Think about the technical flex from the reader's perspective. If it's done up front, you will lose them or scare them off, but if you use it as your project closer, this is what happens...
Wow, look at Alex's work! They are so good at communicating the problem, the findings, visualizaing the data... AND they have this serious technical skill too?? Wow, this person is the total package! We need people like this one the team!
Now that you know how to build a great portfolio, the next step is to make sure it gets seen, which we will cover in the next section.
6. Get good at marketing your skills
You have to learn the skills you'll need for the job, AND learn how to market yourself effectively.
Either one alone is not going to cut it if your goal is to land an Analytics job in 2022.
The first thing you need to do is make sure you look good online when recruiters find you.
What's their first stop? A LinkedIn search for your name.
If you don't show up, that's bad.
If you look like someone who "isn't a data person", that's worse.
Your goal here is that when a recruiter finds your LinkedIn profile, it screams 'Data Person'.
If you don't pass this test, you're not going to get very far.
The best way to explain how to do this well is to just show you a couple of great examples:
Check out Clay Cooper's Profile
I'm using Clay as an example again, because he's someone who did a great job marketing himself. You can see that every inch of his LinkedIn profile screams 'Data Person'.
His profile photo looks professional and friendly
The background image area is filled with relevant credentials
He uses his Featured section to showcase a project portfolio
He's listed a ton of data course credentials under licenses and certifications
When a recruiter is interested in Clay for a data role, and then finds his profile, they become really interested. This is your goal. No need to reinvent the wheel. Just copy his playbook.
Let's do one more example...
Check out Colleen Wright's profile
Colleen was one of our bootcamp students who graduated mid-December, and she is actually starting her new Analyst role today!
Pretty much all of the same things we listed as positives from Clay's profile can be said about Colleen... she looks friendly, she uses the background image area effectively, she creates a project portfolio with her Featured posts section, she has a long list of data-related credentials in her Certifications section.
Look at these profiles. Then look at yours. Be objective. Who do you think a recruiter for an Analytics role would be more excited to hire?
Polishing your LinkedIn profile needs to be your first stop.
This is a place where you may get discovered, and it's also a guaranteed checkpoint during any hiring process. When you do get hired, someone from the company will have looked you up on LinkedIn first to make sure you seem like a fit. This happens before they invite you for an interview. Make sure you aren't filtering yourself out with a weak profile. Let this be a major asset for you.
Okay, now that you've got yourself looking good online, get active in the online data community.
It's a really welcoming group of people, I promise :)
You've got other learners trying to do exactly what you're doing, educators giving away free content all the time, and groups doing fun challenges to learn together every month.
A lot of times people are hesitant to get involved and to put themselves out there, but with so many stories of this leading to being discovered and new job opportunities, you have to get over that initial discomfort and get active. This is the most effective way to network and it will lead to your best job opportunities.
The easiest way to start is just following people you find interesting on LinkedIn. They put content out there, you read it and learn. Not too scary right?
Do this, and you'll learn what the conversations are like. Pay attention to what they talk about, the types of comments people add on their posts, and then eventually you'll feel comfortable commenting yourself to add value to the conversation. These people you've been following will appreciate it, they'll get to know you, and it will lead to opportunities.
Later, the next comfort level milestone is starting to post on your own. You can share what you're learning, a completed project (which you will add to your Featured post section for use as a project portfolio), or talk about whatever else you think is relevant to branding yourself as a data person.
A couple of tips here...
Be conscious of the overall brand you're building
Don't be negative. Don't "vent" online. No one wants to hire a complainer
Another thing you can do to make this easy is participate in monthly data challenges, where you're given a rich data set, a scenario, and an analysis prompt, and your goal is to analyze and share your findings.
We have seen so many examples of this leading to folks meeting new people who eventually offered them jobs after seeing their work in action.
Maven hosts these once a month (check out our blog or our LinkedIn page) and they're always free to enter. Plus you can even win a prize. There are a couple of other companies that sponsor regular challenges too. It doesn't matter who you go with. Just start doing them. It's a great way to learn and to start building your external brand as a data person.
Next, make sure your resume is up to snuff. I won't spend too much time on that here, but we do have a solid resume guide you can take advantage of:
Next, you'll want to learn how to find jobs and apply effectively.
Here is what I would recommend...
Data-specific Recruiters
Local tech publications
Careers sections of companies where you want to work (follow them too)
Job boards (LinkedIn, Indeed, Monster, etc)
Find jobs you're interested in, and then use LinkedIn to figure out who you know who works there.
You want to network your way in. It is so much more effective than just trying to apply online, where you are 1 of 100+ resumes for any open role. Get a recommendation from someone inside whenever you can.
7. Master the data analyst interview
Again, we've got a solid guide that goes into this in detail, so I won't spend too much time on this one.
Just make sure you understand what Interviewers are looking for, how they will assess these areas, and that you are prepared to shine on all fronts:
Cultural fit
Technical skills (Excel, SQL, R, Tableau, python, etc - varies by role)
Quantitative problem solving ability
General business acumen
Ability to self-learn
Communication
Enthusiasm for the opportunity
If you want more on this, check out the guide below:
Wrapping Up - Write Down Your Plan
If you made it this far, good for you! I hope seeing this roadmap has been helpful.
Now it's time to make a plan and write it down.
Then commit, and get to work.
Don't spend more than an hour on the plan. You just need a rough roadmap. The most important thing is that you start taking action toward your goal.
And like I said before, you don't necessarily need Maven courses to pull off this transition.
If you're someone who is great at planning, will be able to hold yourself accountable, and want to keep your budget to the absolute minimum, you can use this roadmap as a plan and turn to free resources like YouTube for learning.
If you're someone who wants a little more structure about what to learn in a comprehensive and logically ordered way, while still keeping your budget pretty low, then pick a solid online course provider and get going. It doesn't have to be Maven. Just make sure it's a provider with a strong reputation. There is a wide range of quality out there.
If you're looking for "been there, done that" professionals to guide you through every step, be hands on with your learning, teach you how to market yourself, help you with interview prep, and connect you with their network, then you probably want to go for an intensive bootcamp. Just keep in mind, some of these go for $10,000+ USD, and like the self-paced courses, quality varies.
For those of you who are interested in Maven's updated Intensive Learning Cohorts, you can learn more below:
READ MORE ABOUT COHORT LEARNING
Whatever path you want to take, and whatever your learning style is, you can succeed in 2022. All you have to do is make your plan, and commit to putting in the work.
Ready to get started
Sign up for FREE today and level up your data skills
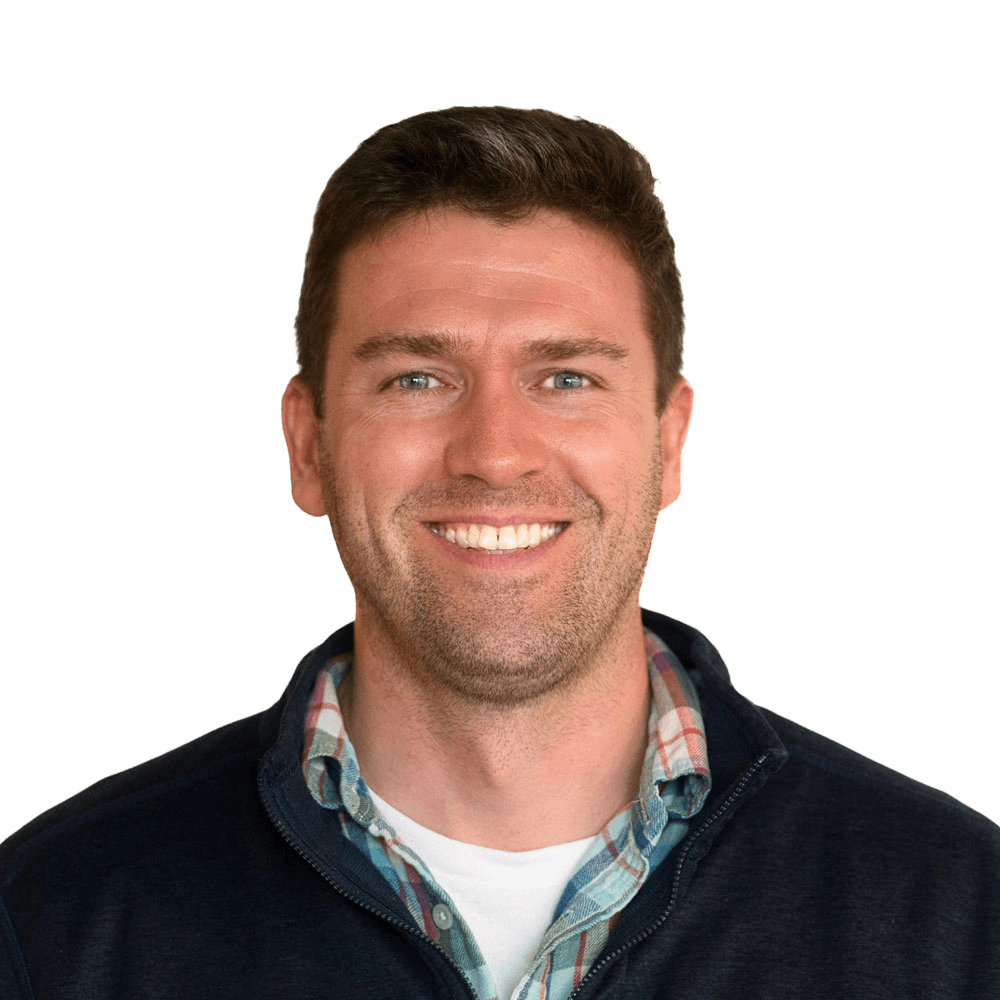
John Pauler
Partner & CRO
John brings over 15 years of business intelligence experience to the Maven team, having worked with companies ranging from Fortune 500 to early-stage startups. As a MySQL expert, he has played leadership roles across analytics, marketing, SaaS and product teams.