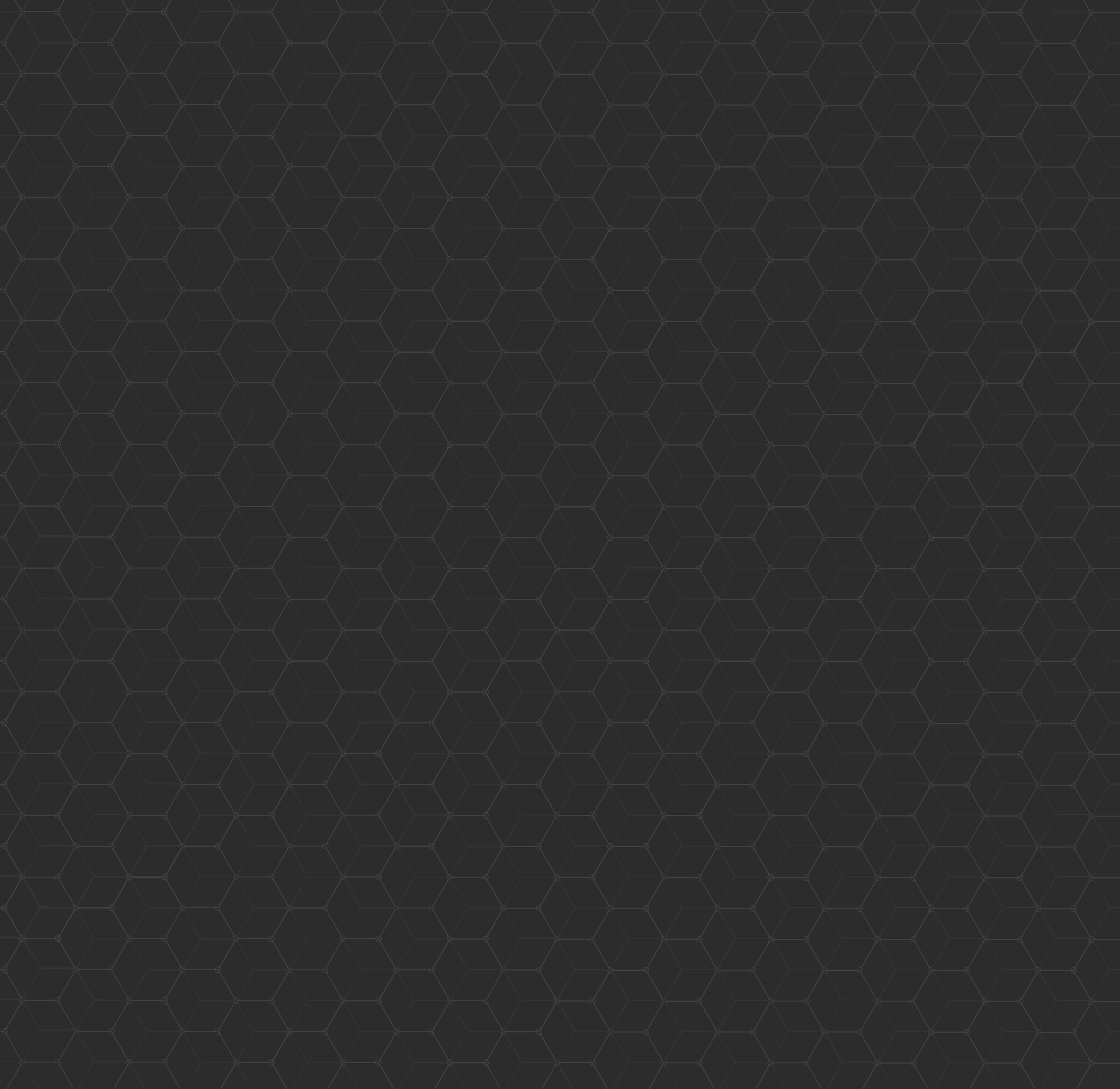
Self-Paced Course
Machine Learning 1: Data Profiling
Explore and prepare raw data for machine learning, and apply a range of univariate & multivariate data profiling techniques.
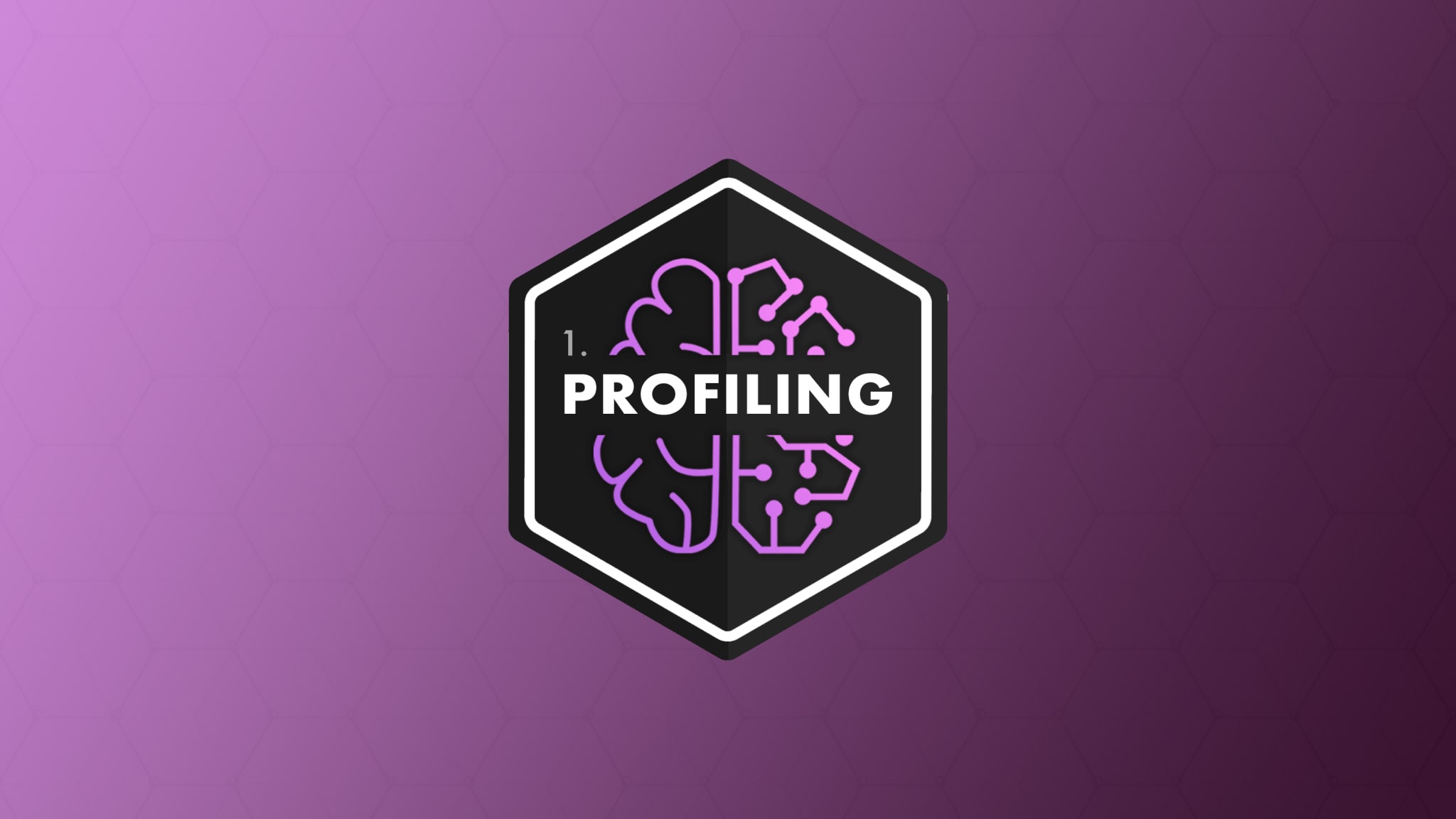
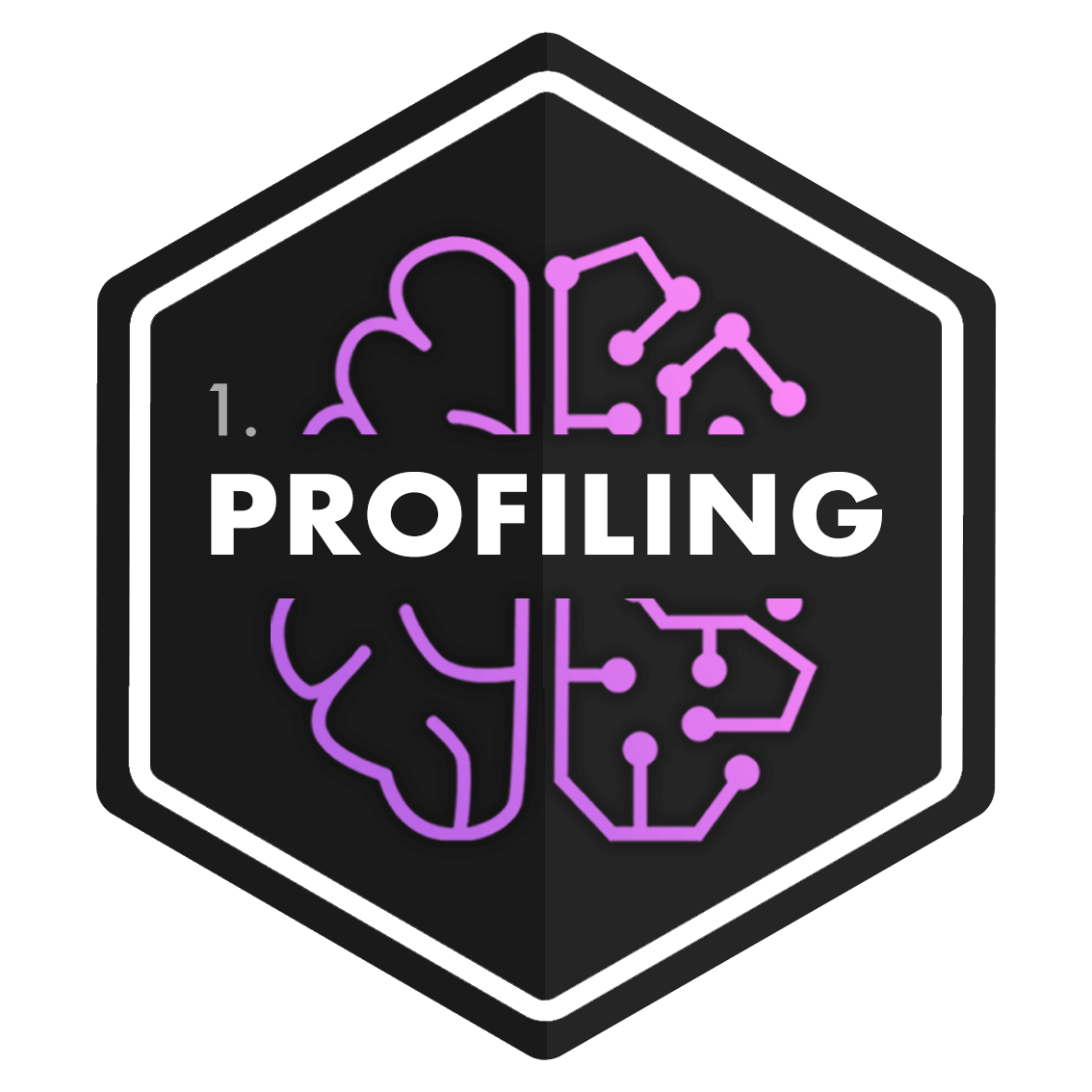
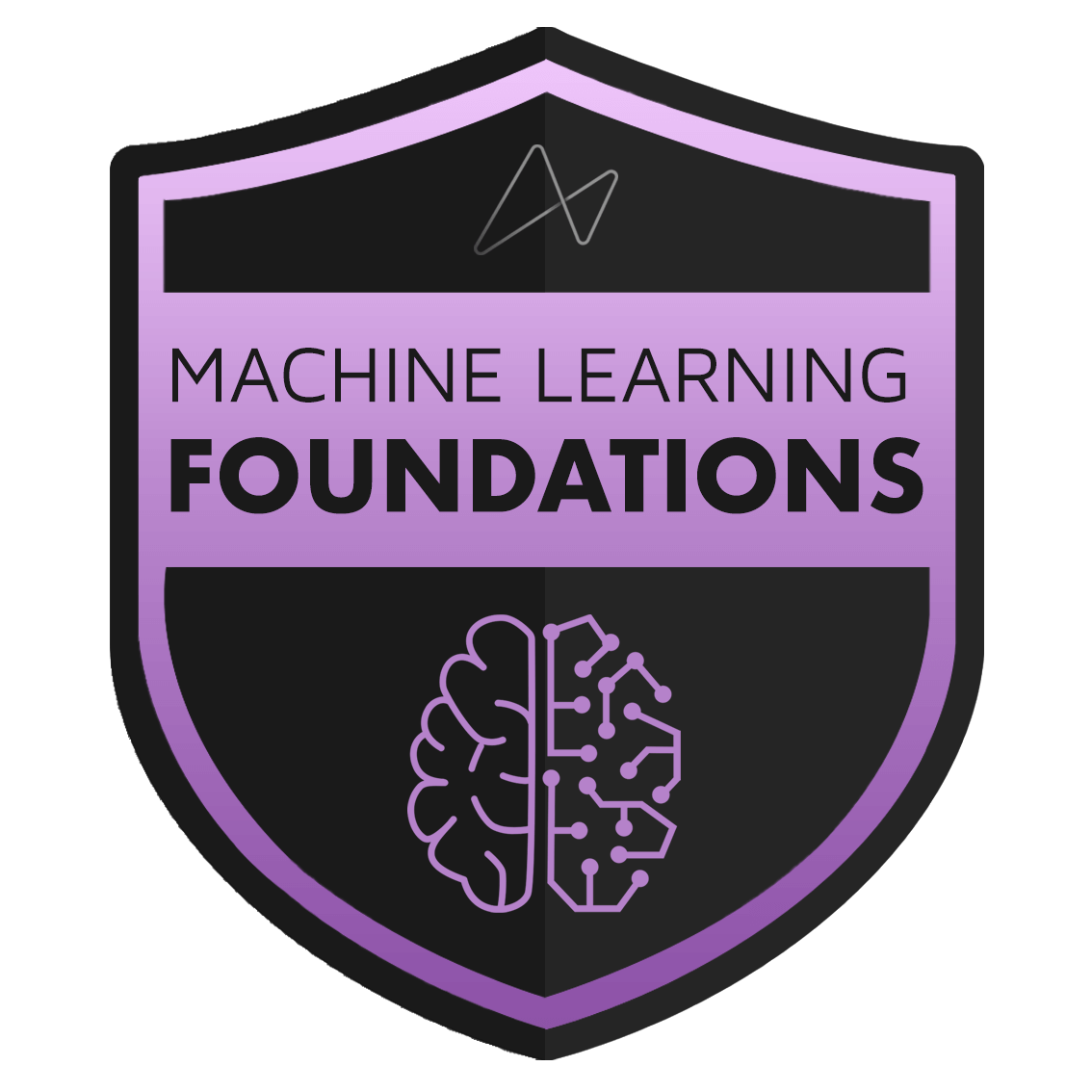
Course Description
This course is PART 1 of a 4-PART SERIES designed to help you build a fundamental understanding of machine learning:
- QA & Data Profiling
- Classification
- Regression & Forecasting
- Unsupervised Learning
In this course we’ll introduce the machine learning landscape and workflow, and review critical QA tips for cleaning and preparing raw data for analysis, including variable types, empty values, range & count calculations, table structures, and more.
We’ll cover univariate analysis with frequency tables, histograms, kernel densities, and profiling metrics, then dive into multivariate profiling tools like heat maps, violin & box plots, scatter plots, and correlation.
Throughout the course we’ll introduce case studies to solidify key concepts and tie them back to real world scenarios. You’ll clean up product inventory data for a local grocery, explore Olympic athlete demographics, visualize traffic accident frequency in New York Ciy, and more.
NOTE: This is NOT a coding course, and doesn't cover programming languages like Python or R. Our goal is to use familiar tools like Excel to demystify complex topics and explain exactly how they work.
If you’re ready to build the foundation for a successful career in data science, this is the course for you.
COURSE CURRICULUM:
- Course Structure & Outline
- About this Series
- DOWNLOAD: Course Resources
- Setting Expectations
- Intro to Machine Learning
- When is ML the Right Fit?
- The Machine Learning Process
- The Machine Learning Landscape
- Introduction
- Why QA?
- Variable Types
- Empty Values
- Range Calculations
- Count Calculations
- Left & Right Censored Data
- Table Structure
- CASE STUDY: Preliminary Data QA
- BEST PRACTICES: Preliminary Data QA
- QUIZ: Preliminary Data QA
- Introduction
- Categorical Variables
- Discretization
- Nominal vs. Ordinal
- Categorical Distributions
- Numerical Variables
- Histograms & Kernal Densities
- CASE STUDY: Histograms
- Normal Distribution
- CASE STUDY: Normal Distribution
- Univariate Data Profiling
- Mode
- Mean
- Median
- Percentile
- Variance
- Standard Deviation
- Skewness
- BEST PRACTICES: Univariate Profiling
- QUIZ: Univariate Profiling
- Introduction
- Categorical-Categorical
- CASE STUDY: Heat Maps
- Categorical-Numerical
- Multivariate Kernal Densities
- Violin Plots
- Box Plots
- Limitations of Categorical Distributions
- Numerical-Numerical
- Correlation
- Correlation vs. Causation
- Visualizing Third Dimension
- CASE STUDY: Correlation
- BEST PRACTICES: Multivariate Profiling
- Looking Ahead
- QUIZ: Multivariate Profiling
- Course Feedback Survey
- Share the love!
- Next Steps
WHO SHOULD TAKE THIS COURSE?
Data Analysts or BI experts looking to transition into a data science role or build a fundamental understanding of core ML topics
R or Python users seeking a deeper understanding of the models and algorithms behind their code
Anyone looking to learn the basics of machine learning through hands-on demos and intuitive, crystal clear explanations
WHAT ARE THE COURSE REQUIREMENTS?
- We'll use Microsoft Excel (Office 365 Pro Plus) for demos, but you are not required to follow along
Start learning for FREE, no credit card required!
Every subscription includes access to the following course materials
- Interactive Project files
- Downloadable e-books
- Graded quizzes and assessments
- 1-on-1 Expert support
- 100% satisfaction guarantee
- Verified credentials & accredited badges
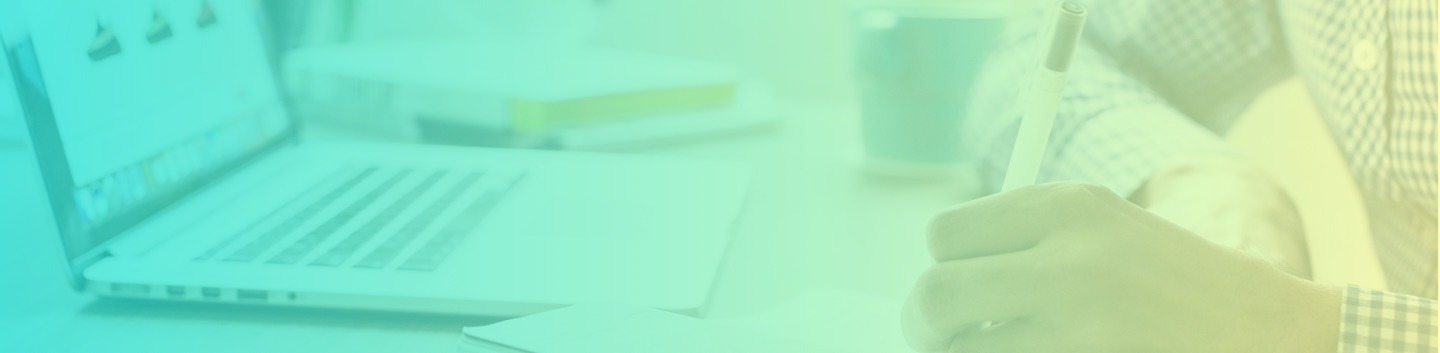
Ready to become a
data rockstar?
Start learning for free, no credit card required!